Motion Plannning of Robot
How can we ensure the safe movement of autonomous systems in a multi-agent environment?"
Navigate robot in multi-agent environment. Ensuring safe, i.e., collision-free, motion for autonomous agents is an ongoing and challenging task. We proposed a safety-critical control framework for an ego agent moving among other agents. The approach infers the dynamics of the other agents online and incorporates the inferred quantities into the design of control barrier function (CBF)-based controllers for the ego agent. The inference method combines offline and online learning with radial basis function neural networks (RBFNNs). Additionally, we employ conformal prediction to quantify the estimation error of the RBFNNs for the other agents’ dynamics, generating prediction intervals to cover the true value with a user-defined confidence. Finally, we formulate a quadratic program (QP)-based controller for the ego agent to guarantee safety with the desired confidence level by accounting for the prediction intervals of other agents’ dynamics in the sampled-data CBF conditions.
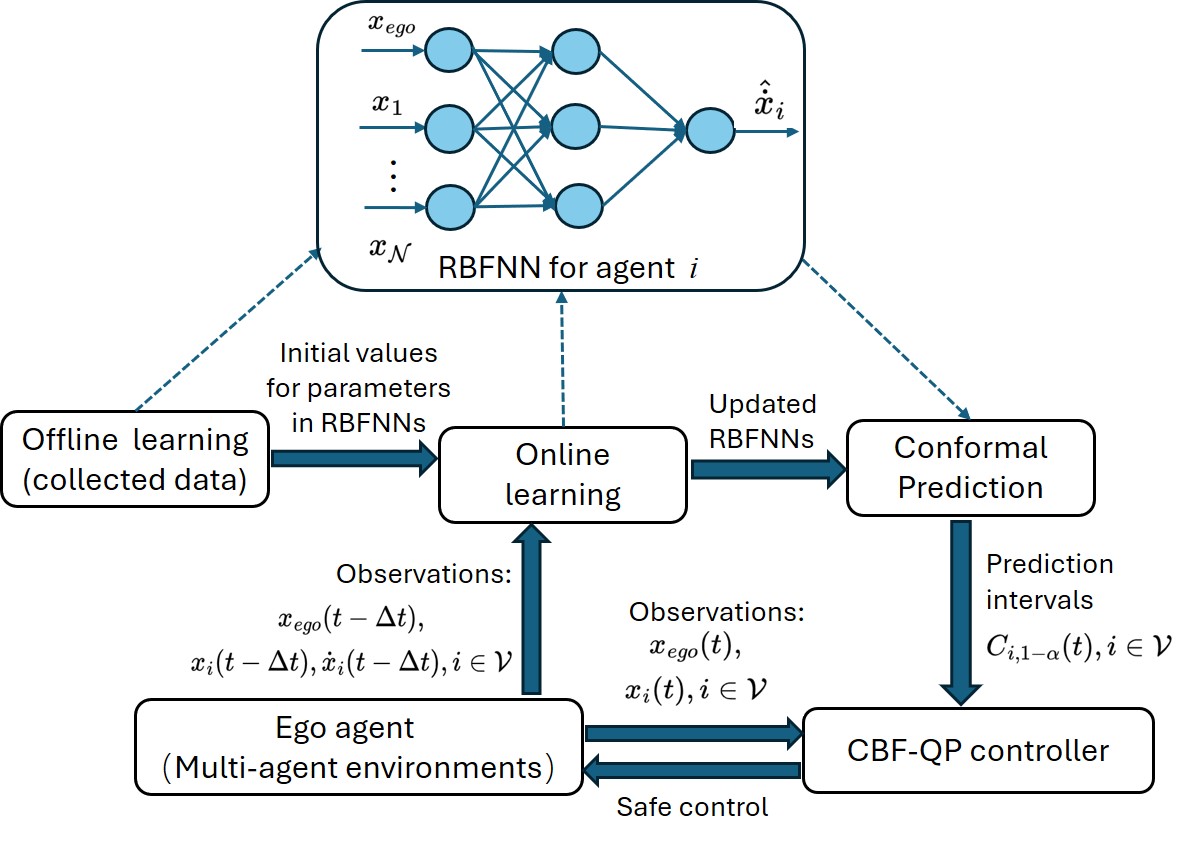